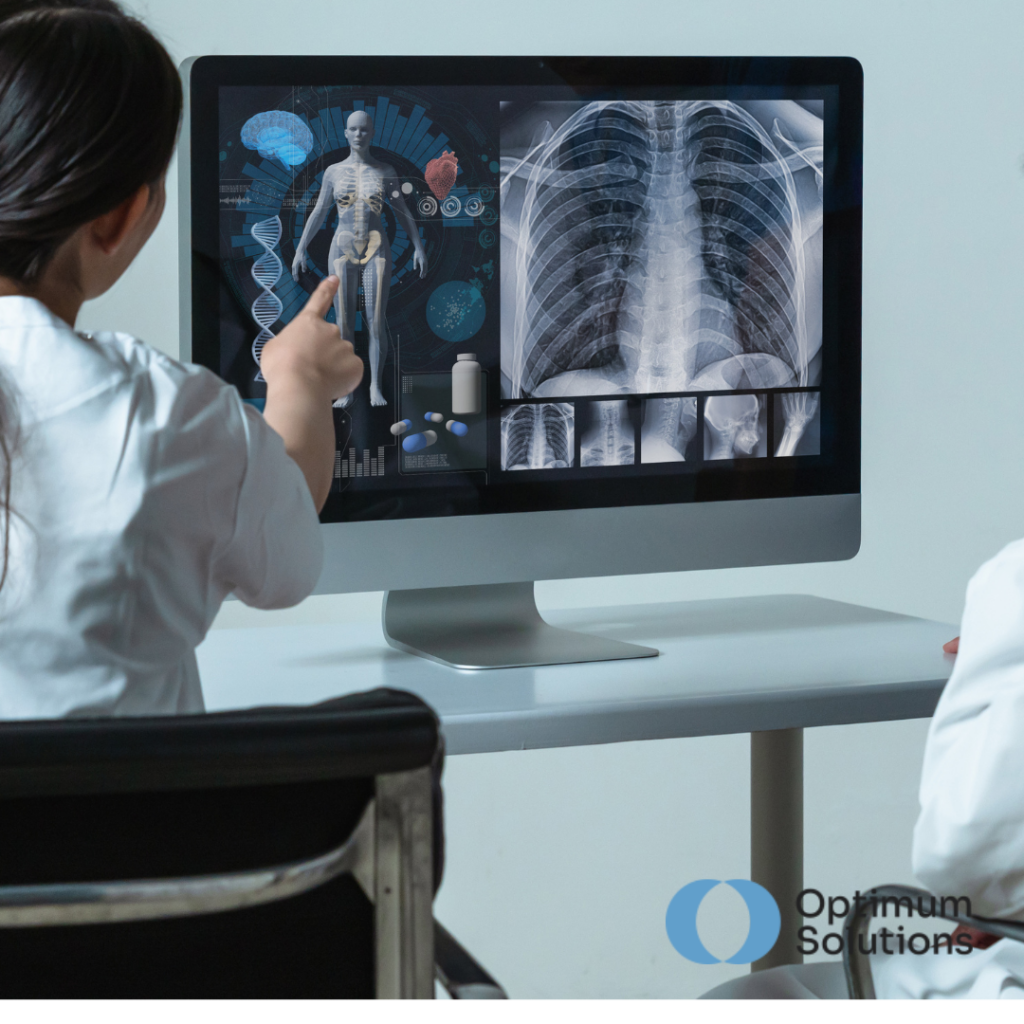
Medical annotation is a critical process in healthcare and various research areas involving meticulously labeling and categorizing medical data. This data can range from clinical notes and patient records to imaging studies and genomic sequences. The importance of medical annotation cannot be overstated, as it plays a pivotal role in improving patient care, advancing medical research, and enhancing the efficacy of machine learning models and AI systems in the medical field.
What is Medical Annotation?
Medical annotation is the process of labeling or tagging medical data such as images, text, and signals with relevant information that can be used for research, analysis, or the development of machine learning models. This process involves identifying and categorizing specific features within the data, such as anatomical structures, pathological conditions, or key clinical terms. The annotated data serves as a crucial foundation for various applications, particularly in healthcare AI, where accurate and consistent annotations are vital for training and validating algorithms.
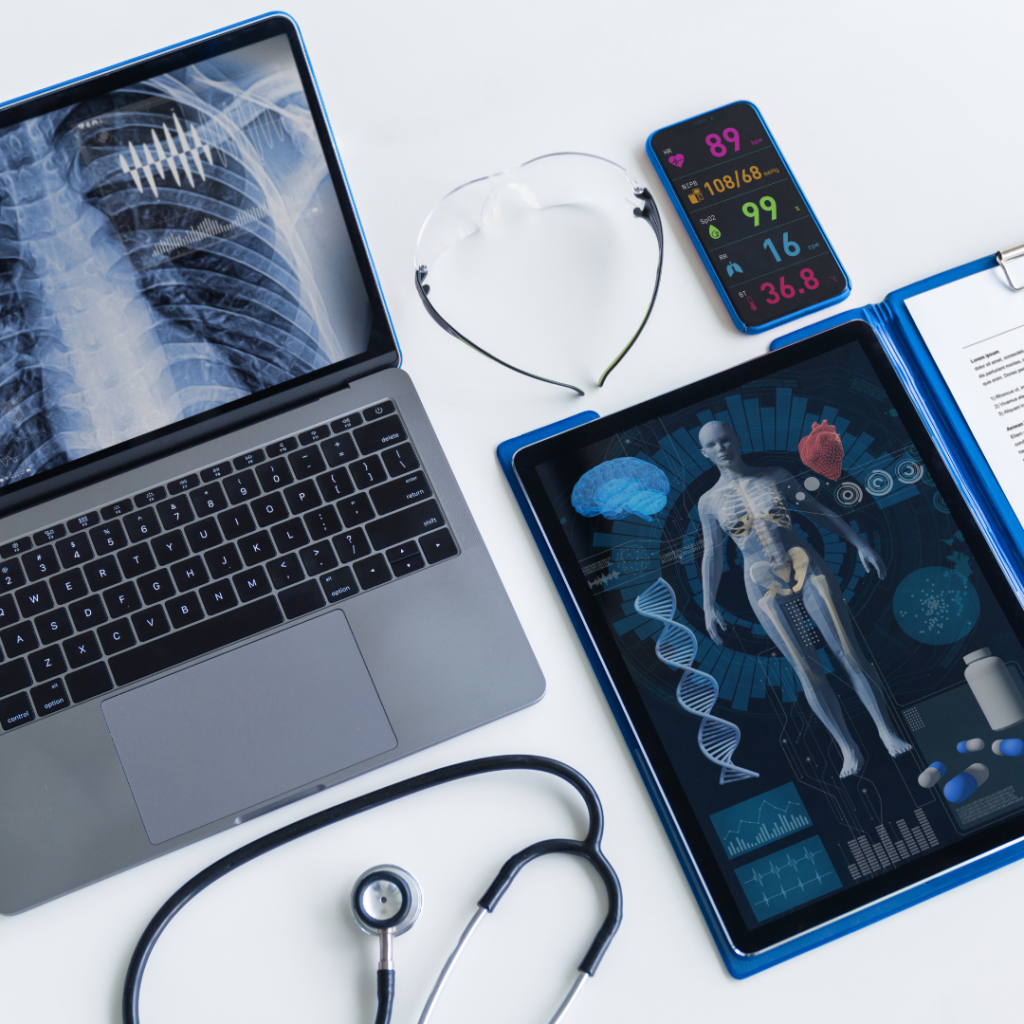
TYPES OF MEDICAL DATA ANNOTATION
- IMAGE ANNOTATION
Bounding Boxes:
- Definition: Bounding boxes are rectangular frames drawn around an object of interest in a medical image to highlight its presence and location.
- Applications: Commonly used in radiology and pathology for tasks such as identifying tumors, fractures, or anomalies in X-rays, CT scans, MRIs, and histopathological images.
- Significance: Bounding boxes are particularly useful in training object detection algorithms, which can automate the identification of specific regions of interest in large volumes of medical images.
Segmentation:
- Definition: Segmentation involves the precise delineation of the boundaries of an object or region of interest within an image. This can be done at a pixel level, creating a detailed outline of structures such as organs, lesions, or pathological areas.
- Applications: Used extensively in medical imaging for the detailed analysis of structures like tumors, organs, blood vessels, and tissues. For example, segmenting the liver in an abdominal CT scan or identifying the exact boundaries of a brain tumor in an MRI.
- Significance: Segmentation is crucial for volumetric analysis, surgical planning, and the development of AI models that require detailed spatial information.
Landmarking:
- Definition: Landmarking involves placing specific points on notable anatomical features within a medical image. These points serve as reference markers for anatomical structures or pathological areas.
- Applications: Used in orthodontics, craniofacial surgery, and biomechanics to identify key landmarks like the corners of the eyes, the tip of the nose, or the center of a lesion.
- Significance: Landmarking is essential for aligning images from different modalities, comparing anatomical changes over time, and facilitating precise surgical interventions.
Polygonal Annotation:
- Definition: Polygonal annotation involves outlining an object or region of interest with a series of connected points, forming a polygonal shape. This method provides more accuracy than bounding boxes.
- Applications: Commonly used in cases where the region of interest has an irregular shape, such as tumors, anatomical structures, or wounds. For example, delineating the contour of a tumor in breast imaging.
- Significance: This technique is particularly valuable for complex anatomical structures, enabling more accurate AI training and better clinical decision support.
2. TEXT ANNOTATION
Entity Recognition (Named Entity Recognition, NER):
- Definition: Entity recognition involves identifying and tagging specific terms or phrases within the clinical text that correspond to predefined categories such as diseases, symptoms, medications, procedures, and anatomical terms.
- Applications: Used in electronic health records (EHR) to extract relevant clinical information, in literature mining for research purposes, and in the development of clinical decision support systems (CDSS).
- Significance: Accurate entity recognition is essential for structuring unstructured clinical data, improving the retrieval of patient information, and enabling better analysis of clinical outcomes.
Relation Extraction:
- Definition: Relation extraction involves identifying and annotating the relationships between different entities within the text. For example, linking a medication to its corresponding disease or connecting a symptom to a diagnosis.
- Applications: Used in the development of NLP models that can automatically generate patient summaries, predict disease progression, or recommend treatments based on the relationships extracted from clinical notes.
- Significance: Relation extraction enhances the understanding of the context within clinical text, supporting more informed decision-making in healthcare settings.
Sentiment Analysis:
- Definition: Sentiment analysis in medical annotation involves labeling parts of the text based on the sentiment conveyed, such as positive, negative, or neutral. In a medical context, this might relate to patient feelings, treatment outcomes, or clinician opinions.
- Applications: Used to gauge patient satisfaction, analyze clinician notes for signs of concern or optimism, and monitor the emotional tone of patient communications in telemedicine.
- Significance: Sentiment analysis can provide valuable insights into patient well-being, clinician attitudes, and the overall emotional climate within healthcare interactions.
Concept Normalization:
- Definition: Concept normalization involves mapping various terms or phrases to a standardized set of concepts or codes, such as those found in medical ontologies like SNOMED CT, ICD-10, or MeSH.
- Applications: Used to standardize the language in clinical documentation, facilitating better interoperability between systems, enhancing data sharing, and improving the consistency of clinical information.
- Significance: Concept normalization is critical for ensuring that different terms referring to the same medical concept are treated uniformly, enabling more accurate data analysis and reporting.
3. SIGNAL ANNOTATIONS
Event Marking:
- Definition: Event marking involves annotating specific events or points of interest within a continuous physiological signal. These events might include arrhythmias in ECG signals or seizure activity in EEG recordings.
- Applications: Used in cardiology to identify irregular heartbeats, in neurology to detect epileptic seizures, and in sleep studies to mark periods of REM or non-REM sleep.
- Significance: Event marking is vital for diagnosing conditions that manifest as brief, transient events within longer physiological recordings. It helps clinicians pinpoint critical moments that may require intervention.
Waveform Segmentation:
- Definition: Waveform segmentation involves dividing continuous physiological signals into distinct segments based on different phases or states of a biological process. Each segment is labeled according to the phase it represents.
- Applications: Used in analyzing ECG signals to segment and label different parts of the cardiac cycle (P wave, QRS complex, T wave), in EEG to segment sleep stages, or in respiratory signals to identify different phases of breathing.
- Significance: Accurate segmentation of waveforms is essential for detailed physiological analysis, helping to understand normal and pathological patterns in patient data.
Peak Detection:
- Definition: Peak detection in signal annotation involves identifying and marking the highest points (peaks) in a physiological signal. These peaks often correspond to significant biological events, such as heartbeats in an ECG.
- Applications: Used in cardiology to analyze heart rate variability, in respiratory studies to measure breathing patterns, and in neurology to detect spikes in brain activity.
- Significance: Peak detection is critical for quantifying physiological rhythms, assessing the regularity of biological processes, and identifying abnormalities that may indicate disease.
Artifact Annotation:
- Definition: Artifact annotation involves identifying and labeling sections of a physiological signal that are distorted or corrupted by noise, movement, or other non-biological factors.
- Applications: Used in the preprocessing of EEG, ECG, or other physiological signals to remove or account for artifacts that could skew analysis results.
- Significance: Properly annotating and handling artifacts ensures the integrity of signal analysis, reducing the risk of misinterpretation due to noise or other non-relevant factors.
Video Annotations
- Definition: Action annotation involves labeling specific surgical actions or maneuvers within the video, such as cutting, suturing, or stapling.
- Applications
- Surgical Training: Annotating actions helps in creating training modules for surgeons, allowing them to study each step in a procedure.
- Robotic Surgery: In robotic-assisted surgeries, action annotations are used to develop and refine algorithms that assist with or automate certain tasks.
Quality Assessment: Reviewing annotated actions helps in assessing the surgeon’s performance, identifying deviations from standard protocols, or recognizing areas needing improvement.
Significance: Action annotation is critical for understanding the nuances of surgical procedures, enabling the creation of detailed instructional content, and supporting AI systems in recognizing and executing surgical tasks.
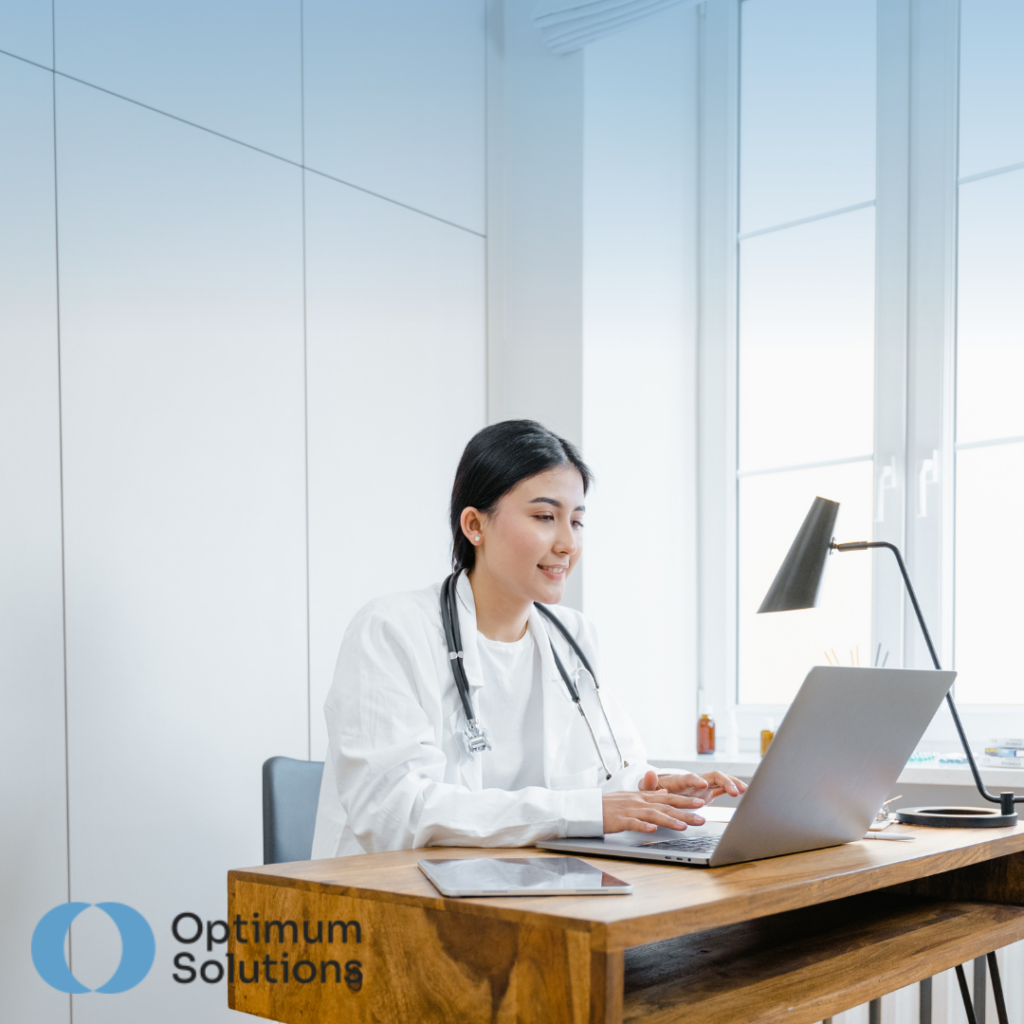
Medical annotation is a foundational element in the integration of data-driven approaches in healthcare and research. Transforming raw medical data into structured and meaningful information enables better clinical decision-making, accelerates medical research, and enhances the development of advanced machine-learning models. As technology continues to evolve, the scope and impact of medical annotation will undoubtedly expand, paving the way for significant advancements in the medical field.
For more information, contact us at luc@optimumsolutions.eu or schedule a virtual coffee chat at https://lnkd.in/eB3QUG8k.